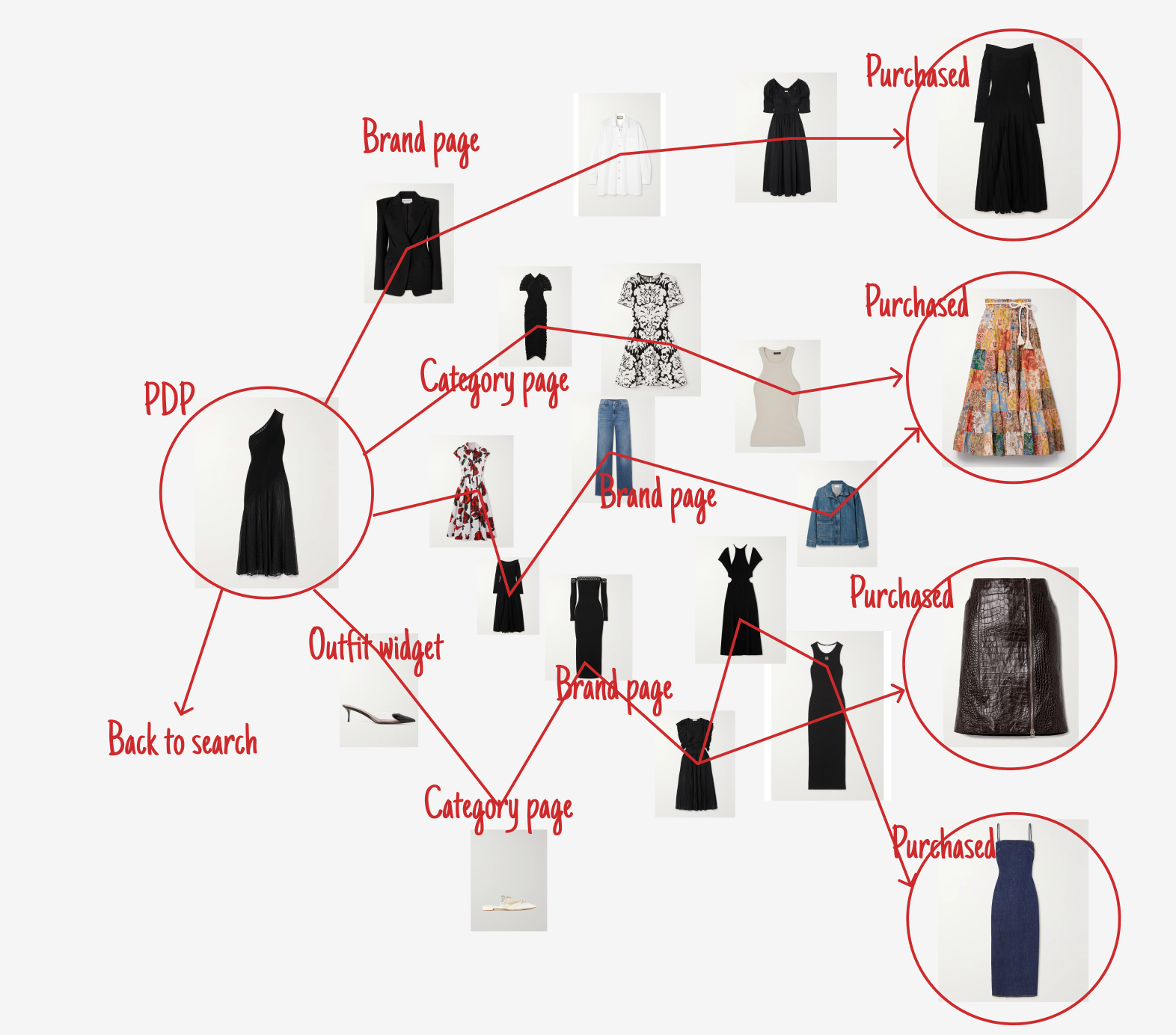
Recommendations
-
Problem
Net-a-Porter features a “You May Also Like” tool on the detail page which makes suggestions based on matching rules provided by the Merchandisers. The conversion rate of this space was very low.
-
Specification
The suggestions provided by the rules weren’t aligning with the customers, so we need alternative options for suggestions
-
Solution
Rather than suggesting products that might be similar for the customers, I thought we could instead explore customer behaviour to generate suggestions of what is likely to be purchased instead
Finding and Refining Recommendations
Rather than finding products that we think a customer might buy, I thought to instead look at the products they actually did buy.
By exploring customer journey data, we could see which products customers ended up buying when browsing on-site. This allowed to be work with the Data Science team to build a propensity to buy model based on whether a product was dropped, added to a wishlist or added to basket.
What was discovered was that customers browse a high variety of products so they may browse for a black dress, but may end up purchasing white socks.
Results
Increased add to bag rate for recommendations from the propensity to buy model vs rules based (+22% vs control)
Notes: the propensity to buy model requires customer engagement to generate behaviour based recommendations - so 100% of recommendations cannot be generated by this model but due to high traffic, this is available within 2 weeks